Membership Warehousing: Future of Supermarkets?
In the modern retail landscape of China, a remarkable shift has taken place within Walmart's business strategy, particularly in the dichotomy between its traditional stores and the rapidly proliferating Sam's Club membership model. As we witness a series of traditional Walmart outlets shutting down, the Sam's Clubs are opening at an unprecedented rate. With a staggering member base of 5 million, Sam's Club rakes in approximately 1.3 billion yuan annually just from membership fees alone. This strikingly different trend raises a pivotal question: could the warehouse membership model represented by Sam's Club ultimately eclipse traditional supermarkets, establishing itself as the dominant force in the retail industry?
Traditional supermarkets appear to be at a crossroads, struggling to adapt to the changing market dynamics. The success of the Sam's Club model stems from a complex interplay of factors rooted in evolving consumer behaviors and preferences. Not too long ago, Carrefour stood as a formidable contender in China’s retail space. Its operations remained largely unchanged from 1995 to 2010, thriving in an era before the explosive growth of online shopping. However, the advent and rapid expansion of e-commerce have fundamentally transformed the shopping choices available to consumers, drastically reducing the once-immutable position of traditional supermarkets.
Advertisement
The comfort and convenience of online shopping have eroded the appeal of physical stores. Consumers can now simply click a mouse or swipe a screen to order a variety of products, often enjoying home delivery services that render the analog shopping experience increasingly obsolete. This shift represents an unprecedented challenge to the traditional supermarket model, compelling businesses to reassess their value propositions.
Simultaneously, the consumer demographic has undergone a profound transformation. Take the 1990s, for example, when activities such as bowling, consuming fast food, and listening to music on portable cassette players epitomized the popular leisure and consumption culture. Fast forward to the present, and many of those past trends have faded. Nowadays, soda is often viewed as the drink of choice for couch potatoes, while KFC is humorously likened to a large restroom, and bowling has become an unfamiliar pastime to younger generations.
The once-dominant middle class, characterized by its enthusiasm for color television, vinyl records, and dining out, has evolved. Today's middle-class individuals are more inclined to engage in suburban camping trips, sport smartwatches like the Apple Watch, savor haute cuisine like omakase, and wear high-end outdoor apparel brands like Arc'teryx. This new consumer segment is fiercely focused on elevating their lifestyle, desiring premium quality that communicates their socio-economic status, often through consumption behaviors that establish a sense of exclusivity.
This need for differentiation is brilliantly catered to by the Sam's Club operational model. With a membership fee of 260 yuan, it sets an initial barrier that segregates the consumer pool. Shopping within Sam's Club often requires spending thousands of yuan, presenting a financial threshold that not all typical consumers can meet. The club's allure lies in its association with affluence and exclusivity—attributes that resonate well with the aspirational middle class.
The logistical demands of shopping at a Sam's Club require consumers to own vehicles capable of transport and sufficient storage space at home for large quantities of goods. These hurdles inadvertently become a badge of honor for those within the middle class, often shared through social media platforms like Xiaohongshu or WeChat, as they curate the narrative of their quality of life.
To add to its attractiveness, Sam's Club possesses solid foundations that resonate with discerning consumers. Middle-class shoppers are savvy and not easily swayed by mere marketing gimmicks; thus, Sam's Club strategically imposes membership fees while ensuring that its product quality justifies the costs. The club’s rigorous standards allow it to deliver exceptional products consistently, a promise that is upheld through its comprehensive supply chain framework.
Boasting over 4,000 SKUs, Sam's Club's robust supply chain is further supplemented by backing from Walmart, the retail giant itself. This relationship grants Sam's a unique advantage in navigating market demands, particularly within the fast-evolving categories of pastries, deli items, and fresh produce—segments that have recently become battlegrounds in supermarket competition. Unlike many of its competitors, which struggle to fend off the popularity of online shopping, Sam's Club focuses on fresh-baked goods and ready-to-eat items that in-store consumers inherently prefer due to freshness and quality guarantees.
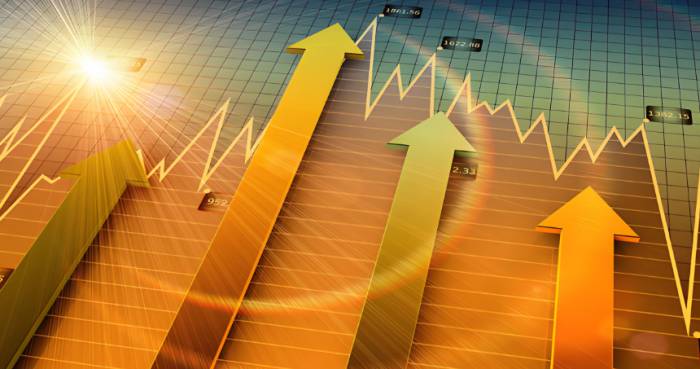
Moreover, the private-label Member's Mark brand adds another layer of appeal. With over 700 unique products, it accounts for more than 35% of the club's marked inventory, showcasing a brand built through decades of Walmart's savvy global supply chain management. This depth of experience enables them to deliver consistently high-quality products at competitive prices, a feat that new entrants into the market find challenging to replicate.
Contrastingly, several retailers have attempted to emulate the membership model without understanding the underlying strategic importance of supply chain capabilities. Carrefour made a recent pivot towards a membership strategy but continues to struggle amidst faltering sales, illustrating that mere imitation without substantive supply chain vision is a path to failure. The critical question then becomes whether the warehouse membership model will replace traditional supermarkets. Each operates within the same offline retail domain but caters to different market segments and consumer preferences.
Just as department stores maintain their relevance within the retail landscape, it is conceivable that a diverse array of retail formats can coexist, each serving specific consumer needs. A balanced mix of retail formats will ultimately depend on the preferences and purchasing behavior of the consumer base. With the rise of the middle class and their burgeoning purchasing power, warehouse membership models are poised for rapid expansion, continually innovating and integrating strategies, much like Hema, which adopts membership concepts while tailoring offerings to the unique tastes of Chinese consumers, including specialty items and boutique selections catering to contemporary preferences.
Comment