New Changes in Inventory Cycles
Understanding the Dynamics of Inventory Trends in 2024
In recent economic discussions, a pivotal question has surfaced: Why has the growth of inventories begun to decline rapidly after a six-month period of steady improvement? This inquiry is essential, as inventory management serves as a vital indicator of economic health and stability. At the heart of this phenomenon lies the intricate relationship between corporate profitability, inventory levels, and the current demand landscape.
Recent analysis has shown that corporate profitability often leads inventory cycles, with a historical lag time of about three to four quarters that has significantly compressed in 2022 to a mere two to three quarters. This means that when businesses see a rise in profit, it does not take long before those improvements begin influencing inventory levels. For instance, in June 2023, a new phase of profit improvement for industrial enterprises was identified, suggesting a possible upturn in inventory levels around early 2024. However, recent data indicates that since August of this year, the rate of recovery in corporate profits has begun to slow down, which may suggest a corresponding reduction in the momentum for restocking inventories down the line.
Moreover, it's noteworthy that the nominal velocity of inventory growth has significantly declined in recent months largely due to pricing pressures. For example, nominal inventory growth had been steadily increasing for eight months before entering a downward trend in the last three months, illustrating how prices play a critical role in inventory liquidation. The Producer Price Index (PPI) has seen a marginal decline which directly impacts nominal inventory levels. Removing price factors tells a different story, with actual inventory growth also slowing down, revealing a deeper issue: lackluster profit growth is severely hindering restocking impulses.
Advertisement
Weak domestic demand has exacerbated profit drops, consequently leading to greater inventory depletion, further influenced by an elevated inventory-to-sales ratio. As the year progresses into 2024, industries heavily reliant on domestic demand have experienced notable declines in both actual revenue and profit growth rates, much more significant than those reliant on export markets. This shows that declining domestic demand is a primary catalyst for the inventory liquidation trend observed in many sectors. In tangible terms, by October 2024, the inventory-to-sales ratio in domestic-demand-dependent industries had risen to 0.47, reinforcing the narrative of active inventory depletion.
Sectoral Divergence in Inventory Trends
Exploring the structural dimensions of inventory dynamics, it becomes clear that different sectors are experiencing notable divergences in inventory trends. Since the latter half of 2023, sectors such as processing and manufacturing have been actively restocking, in contrast to consumer goods manufacturing, which persists in inventory depletion. Several characteristics arise when examining sectoral performance in inventory management. For one, the inventory growth rate in processing and manufacturing sectors is highly sensitive to price fluctuations, especially when real inventory counts are analyzed apart from pricing shifts. Furthermore, since the middle of 2023, there has been a significant recovery in inventory for these industries, with actual inventory levels rebounding markedly from their lowest points.
In light of supportive policies introduced in late 2023 aimed at bolstering economic growth—such as financial stimulus packages—the manufacturing sector's revival has been palpable. This has been coupled with a natural cycle of equipment renewal, helping to sustain high levels of manufacturing investment. Results are observable within the non-metallic mineral products and general equipment manufacturing sectors, which have shown marked increases in inventory growth since mid-2024, rising significantly from lows earlier in the year. Yet against this progress, the consumer goods manufacturing sector is still struggling to rebound from diminished inventory levels.
As we look to the remaining months of 2024, we must address the disparities in consumer demand uptake compared to investments. During the period between September 2023 to June 2024, consumer demand growth has not kept pace with investment growth, as evident in the diminishing central growth trend for retail and tangible declines in specific consumer goods sectors, such as food and beverages. Real inventory growth in the consumer sector has slowed to a ten-year low, underscoring significant challenges as firms focus on reducing inventory instead of replenishing it.
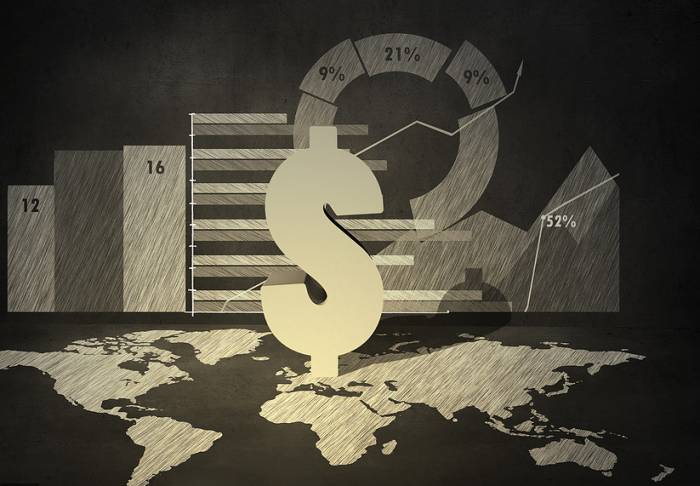
Furthermore, the reverberating effects of falling commodity prices have considerably affected the processing and manufacturing sectors. From July to October 2024, the notable decline in PPI affected inventories directly, with processed materials witnessing a fall in nominal inventory growth of 2.7 percentage points. This impact is distinct from the performance of consumer goods, where nominal inventory levels have paradoxically shown a minor uptick. However, this doesn't alleviate the concern that persistent pricing declines may hinder further inventory recovery efforts within higher-demand sectors.
Future Prospects for Inventory Replenishment
Looking ahead, the sectors poised for inventory replenishment success are those that are able to leverage the "new export" economic strategies that have arisen in response to current profitability pressures. Many sectors reliant on external demand may find themselves in a more favorable position into mid-2024 due to a direct tie to rising export orders. For example, industries such as chemicals and telecommunications equipment have noted significant year-over-year increases in export delivery values, lending crucial support to their profitability. The anticipated increase in export orders serves as a reliable signal of potential inventory recovery based on observed lags in profitability translating into restocking efforts.
In addition, the anticipated implementation of "two new" policies aimed to stimulate domestic demand is likely to further impact sectors related to equipment upgrades and consumer appliance replacements during the latter half of 2024. The appliance and automotive sectors, for instance, have already exhibited recovering sales trends as policies come into effect. Coupled with historically low inventory-to-sales ratios in automotive and electrical machinery sectors, there is significant potential for restocking within these industries throughout the first half of the coming year.
However, while some sectors appear poised for recovery, it's essential to note that low-value consumer goods are experiencing elevated inventory-to-sales ratios, indicating limited scope for replenishment in the near term. Industries such as beverages and food are witnessing stagnant growth rates and increasing pressures with low profitability, which further complicates their ability to manage inventory levels effectively. This combined with mixed capacity utilization rates across supply chains leads to conservative expectations regarding inventory replenishments.
Comment