Non-Bank Interbank Deposit Rate Cut
The recent shifts in interest rates within China's financial markets have sparked considerable conversation among industry experts, particularly concerning the implications for futures companies. As non-bank financial institutions and corporate deposit rates continue to plunge, the potential ramifications for margin interest income at futures firms are becoming increasingly evident.
In a recent commentary, market specialists have posited that these interest rate adjustments are likely to dampen the financial returns of futures companies, necessitating a comprehensive reassessment of their cash management strategies. This adjustment period, they suggest, exudes a sense of urgency, as futures brokers need to respond adeptly to the emerging challenges brought forth by this significant change in the interest rate landscape.
According to data released by the China Futures Market Monitoring Center, there has been a remarkable upswing in the scale of customer equity held by futures companies in recent years, ballooning from around 5 trillion yuan at the end of 2019 to approximately 14 trillion yuan by mid-2024. This growth underscores the importance of effective financial management within the sector.
Impact of Margin Interest Rate Reductions
The analysis conducted by Liao Zhiming, a fixed income analyst at Huayuan Securities, indicates that ongoing policy initiatives have driven interbank demand deposit rates below 1.5%. This shift means that future adjustments will likely align with the fluctuations of the People's Bank of China's (PBOC) seven-day reverse repurchase agreement rates. Liao noted that certain publicly listed futures companies have been operating with deposit rates around 2.0%. Therefore, the recent adjustment to 1.5% could drastically lower the interest income earned on margin deposits, posing substantive threats to the profitability of these firms and potentially altering the overall operational framework of the industry.
Advertisement
This sentiment is echoed by another insider in the futures arena, who elaborated on how futures companies have typically relied on margin deposit interest income as a core revenue stream. To attract more client funds, several firms had previously offered incentives that provided returns exceeding the standard interest rates. This practice effectively inflated short-term asset allocations; however, it raises questions concerning the long-term sustainability and responsible financial practices of these companies.
“With the downturn in non-bank interbank deposit rates, futures firms will face a significant shrinkage in this income source,” the insider commented. While the immediate fiscal pressures on interest income are clear, the broader implications suggest that these market shifts could ultimately lead to an optimization of fund allocations in the financial marketplace, stimulating healthier long-term development. Futures companies must adapt their strategies and earnestly seek new growth avenues amid this transitional climate.
To understand the context better, it’s essential to grasp how margin deposits function within futures trading. Customers entering futures contracts are mandated to pay a proportion of their investment as a margin to the futures company. These margin deposits fundamentally represent a crucial source of capital for futures firms, which typically channel this money into various financial institutions like banks to accrue interest income. The stability and scale of this income are intrinsically linked to the volume of client margin deposits and the prevailing deposit rates.
In a bid to thrive in an intensely competitive market, numerous futures companies have previously leveraged strategies that involved offering additional rebates on the standard 2.0% rates. On a short-term basis, these tactics produced significant results, enabling firms to draw in more deposits from clients eager for higher yields, thereby expanding their asset base rapidly. The appeal of enhanced actual interest returns led to a marked preference among clients for these futures firms, resulting in heightened market share and improved competitive positioning. This influx of available capital inevitably supported the firms' operational growth and capacity to expand their services.
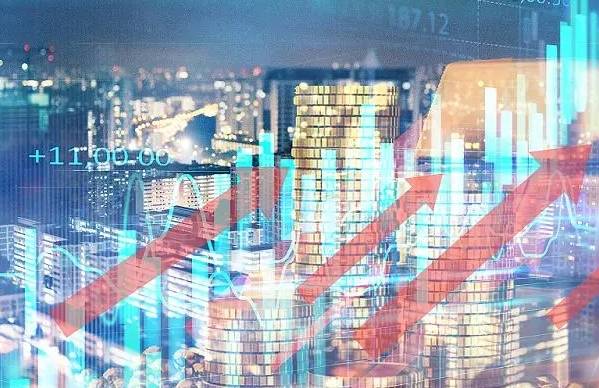
Contraction of Industry Profitability
Entering the current year, futures companies have encountered stark challenges with profitability. During the first half of the year, the dual pressures of altered exchange return policies and intensified sector competition, coupled with the prevailing low-interest-rate environment, have placed substantial constraints on traditional brokerage operations.
Statistics compiled by the China Futures Industry Association reveal sobering figures: from January to June 2024, 150 major futures companies saw their combined revenues dip to 17.976 billion yuan, marking a 3.81% year-on-year contraction. They reported a net profit of 3.844 billion yuan, reflecting a significant 22.45% decline compared to the previous year.
As 2024's final quarter approaches, however, market trading enthusiasm appears to be regaining momentum, resulting in a partial alleviation of profit decline for futures firms. Recent updates from the China Futures Industry Association indicate that between January and October, industry revenues reached approximately 32.763 billion yuan, slightly surpassing the previous year's figures, with net profits totaling around 7.875 billion yuan—down about 2.9% year-on-year.
Industry analysts decipher that the implementation of recent initiatives presents a potential shift in capital flows towards the bond market, which could slightly enhance liquidity for traditional lending sectors. Should regulatory frameworks impose caps on margin rates that futures companies can offer, it could prompt a reallocation of funds away from futures trading and provide some relief to firms' profit margins.
Adding to this narrative, Jing Chuan, the chief economist at Dongya Futures in Shanghai, pointed out that since late September, the Chinese government has introduced a comprehensive suite of policies designed to invigorate stock and related markets. These initiatives have positively influenced trading volumes in the futures sector. Additionally, there has been an uptick in self-investment returns for futures companies, with some interest yields recognized in October contributing to performance growth. However, Jing cautioned that by December, the fervor for market trading might diminish, potentially causing a downturn in both revenue and profit growth across the industry.
Comment