Shifting Sands: U.S. Bonds, Gold, and Stocks
The recent fluctuations in the U.S. markets, particularly in bonds, stocks, and gold, have left many investors perplexed and anxious. As key indicators like the yield on the two-year U.S. Treasury bonds approached 4.32% and the U.S. dollar index rose above 106.90, even as the S&P 500 fell 0.48%, the atmosphere seemed laden with uncertainty. The precious metal gold, often viewed as a safe haven, also suffered a decline of approximately $6, settling around $2570 per ounce. It appears that the market, which had anticipated aggressive rate cuts from the Federal Reserve following recent economic developments, is re-evaluating its stance.
Amidst this backdrop, the Federal Reserve's signals have been anything but dovish, countering traders' expectations for immediate rate cuts. The Fed has been cautious about lowering rates quickly, emphasizing a "gradual" approach rather than the sharp cuts that many had hoped for. This caution has led to a significant reduction in expectations for monetary easing, as traders reassess the likelihood of rate cuts at the upcoming Federal Open Market Committee (FOMC) meetings. Just days ago, market participants foresaw nearly 100 basis points of cuts; now, that number has dwindled to a more tempered expectation of three cuts of 25 basis points before year's end.
Advertisement
Economic data released in recent weeks has not been encouraging, adding fuel to the unease surrounding the outlook for growth. Initial jobless claims for unemployment benefits remain elevated, signaling underlying weakness in the labor market, while existing home sales have only recently shown signs of growth, achieving a rise for the first time in five months amidst continuing record high housing prices. The Chicago Fed's national Purchasing Managers' Index saw a steep decline, reflecting the fastest contraction rate in manufacturing sentiment for the year. This confluence of negative indicators creates a complex web that investors must navigate with caution.
The robust performance of the U.S. economy has been a key pillar supporting the dollar's current strength. Recent economic data shows steady growth and low unemployment rates, enticing both domestic and international investors into dollar-denominated assets. This influx of capital reinforces the dollar's rise; the relative attractiveness of U.S. investments amidst a sea of lower rates abroad fuels demand for the currency. Such dynamics underscore the notion that confidence in the U.S. economy remains strong, even as concerns about growth materialize.
A significant driver of the dollar's rally also stems from the tightening monetary policies orchestrated by the Federal Reserve. As the Fed raises interest rates, the yield on dollar assets becomes relatively more appealing compared to those in countries where interest rates remain stagnant or low. The tangible yields gained by holding U.S. assets entice investors to shift their capital to the States, contributing to the dollar's strength. Moreover, amidst global political and economic uncertainties, the dollar is often viewed as a safe haven—reinforcing its appeal when uncertainties prevail.
BlackRock has commented on the recent fluctuations, suggesting that the sharp decline in U.S. Treasury yields was perhaps a market overreaction rather than a true reflection of underlying fundamentals. They argue that while there might be long-term challenges facing U.S. debt, short-term contractions in the Treasury market may not pose immediate risks to equities. Historical trends have seen periods of high yields not necessarily correlate with stock market declines, and market reconfigurations may be seeking higher compensation for holding bonds amidst evolving economic indicators.
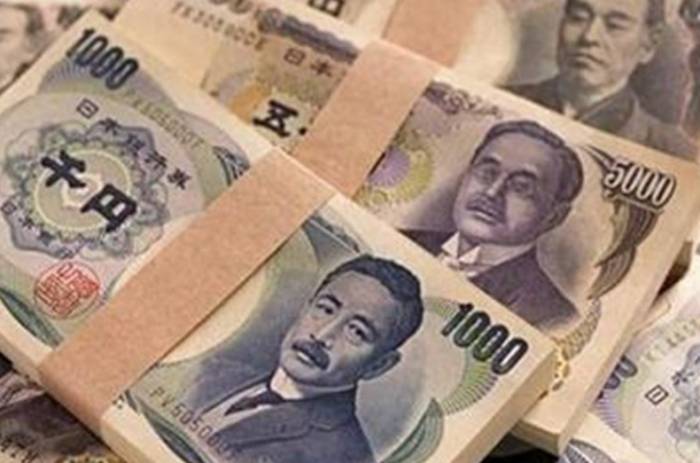
Furthermore, the conflicting performance between bullish economic indicators and bear-like movements in U.S. bonds puzzles many. Even with strengthening economic data, market participants are treating bonds favorably, partly due to geopolitical tensions exacerbating fears of economic instability. The backdrop of rising diplomatic tensions, notably in Eastern Europe and the deterioration of East-West relations, creates a complex interplay between perceived risk and investment allocation. Therefore, market sentiments remain fragile, and the Fed's caution further complicates investors' decision-making landscape.
In the realm of precious metals, gold has also felt the weight of market apprehension, primarily driven by the relentless surge in global yields. With U.S. Treasury rates rising, the opportunity cost of holding non-yielding assets like gold significantly increases. As governmental bonds offer more attractive yields, investors tend to pivot towards those, retreating from gold, which traditionally thrives in low-interest environments. The anticipated rise in rates, exacerbated by expectations of continued inflation, creates a precarious situation for gold, as its draw as a hedge against inflation diminishes amid a rising rate environment.
Geopolitical factors, while often supportive of gold prices, have begun to lose grounding as relief from market tensions remains elusive. Current events in the Middle East, particularly the continuing conflicts between Israel and Hamas, have yet to spark any sustained rallies in gold prices. This stagnation points to a broader sentiment—investors appear increasingly desensitized to geopolitical risks, prioritizing yield-generating investments over classic safe havens. Central banks, particularly in Europe, have signaled possible interest rate hikes as inflationary pressures mount, further diminishing gold's allure.
The U.S. equity markets’ responses have similarly mirrored these dynamics. Investors are assessing the implications of the Fed's evolving rate policies, leading to a reevaluation of high-growth tech stocks, which rely heavily on low-interest rates for expansion. Observations from the recent consumer price index show a year-on-year increase, prompting markets to brace for potential rate hikes that could stifle corporate profits. As detailed earnings reports surface, significant misses in expected growth among key sectors—especially retail—underscore investor fears. Disappointing quarterly forecasts have amplified bearish sentiments, engendering a defensive posture among traders.
Moreover, the landscape is shaped by rising geopolitical risks amidst continuing tensions in Ukraine and U.S.-China relations. As conflict and uncertainty reign across the globe, cautious investors may yield to defensive strategies, opting for stocks in sectors like health care and utilities, which are viewed as more stable during times of economic turbulence. In the face of persistent market volatility and uncertainties surrounding Federal Reserve policy, fluctuations in stock values reflect an underlying fear that any recovery may be short-lived.
Lastly, comments from key figures such as Jerome Powell have further influenced market dynamics, with his pronouncement that there is no rush for rate cuts leading to significant adjustments in market expectations. As the likelihood of immediate rate reductions diminished overnight, the ripple effects across currencies and equities were profound—leading to sudden sell-offs. The volatility of major indices revealed stark implications for sectors highly sensitive to interest rate adjustments, painting a picture of a market wrestling with the complexities of economic reality in the face of policy uncertainties.
Therefore, investors are confronted with a multifaceted landscape that requires diligence and keen insight into economic indicators, monetary policy shifts, and broader geopolitical ramifications. As the U.S. positions itself within these fluctuating global variables, the evolving market conditions will inevitably shape investment strategies and highlight the importance of remaining informed and adaptable in these unpredictable times.
Comment