Leading Enterprises Go Public in Hong Kong
In recent years, Hong Kong has emerged as an attractive destination for mainland Chinese companies looking to list their shares on a global stage. As of late November 2023, a notable trend has surfaced, marked by the surge in the number of esteemed firms transmitting their intentions to go public in the Special Administrative Region. Major players such as Midea Group, China Resources Beverages, and SF Holdings have all opted for listings in Hong Kong, showcasing the city’s enduring appeal as a financial hub.
This movement is significant, with the latest statistics from the China Securities Regulatory Commission revealing that by November 21, a total of 129 domestic enterprises had submitted their applications for overseas listings, of which 97 have specifically chosen the Hong Kong Stock Exchange (HKEX). Such figures are an illustration of the ongoing trend wherein mainland corporations are seeking greater visibility and accessibility to international markets through this platform.
A multitude of favorable policies and streamlined regulations have contributed to the heightened activity in the Hong Kong market, enhancing its attractiveness. With the overall vibrancy of the market now significantly enhanced, Hong Kong continues to be a primary destination for new economic sectors seeking capital and expansion, particularly for those companies rooted firmly in technology and innovation.
Advertisement
Wave of Enterprises Seeking Hong Kong Listings
As 2023 progressed, the buzz surrounding Hong Kong's IPO market intensified. Particularly in the fourth quarter alone, notable companies including Horizon Robotics and China Resources Beverages, among a total of over ten enterprises, successfully made their debut on the HKEX. This trend underscores the market's vitality and its role as a beacon for innovative companies seeking to establish their footprint.
On November 19, SF Holdings announced the commencement of its secondary listing process in Hong Kong, aiming to issue 170 million H-shares globally, with a projected fundraising goal of approximately HKD 5.661 billion. Such moves suggest a strategic endeavor on the part of these companies to diversify their funding sources and reach broader investor bases.
SF Holdings stated in its prospectus that listing as an H-share company would not only enhance its coverage by international investment banks but would also allow for greater engagement with international investors who may not have access to A-shares. This creates a golden opportunity to expand its investor profile.
Beyond giants like Midea and SF Holdings, the landscape includes a variety of consumer-driven companies such as TeaBaidao, as well as technology-focused enterprises like Black Sesame Technologies and Horizon Robotics, indicating a diverse array of sectors finding footing in the Hong Kong market.
To highlight the momentum, Horizon Robotics saw a significant market response when it listed on October 24, achieving a fundraising total of HKD 5.4 billion, marking it the largest technology IPO in Hong Kong for that year. Similarly, on October 23, China Resources Beverages made waves by listing on the HKEX, joining the ranks as the second bottled water company to do so following Nongfu Spring.
Earlier in September, Midea Group celebrated its grand listing day at the HKEX with an IPO that attracted an impressive HKD 30.668 billion, making it the largest public offering in Asia and the second largest globally for the first three quarters of 2023.
According to a report from KPMG, the Hong Kong market had recorded 45 listings by the end of the third quarter, raising a total of HKD 55.6 billion. These statistics have positioned Hong Kong firmly back among the top five global IPO markets.
Professor Xiang Qian from Lingnan College at Sun Yat-sen University noted, "China's innovation landscape is robust and continually flourishing. As many firms seek IPOs to support their growth, Hong Kong has become one of their top choices."
Further, analysts have pointed out that the prevalence of technology-driven enterprises is striking this year, with leading companies representing a large portion of the listings. The openness and international character of the Hong Kong market are enticing for companies wishing to enhance liquidity and attract varied investor types, thereby improving their capital access and securing favorable conditions for sustainable growth.
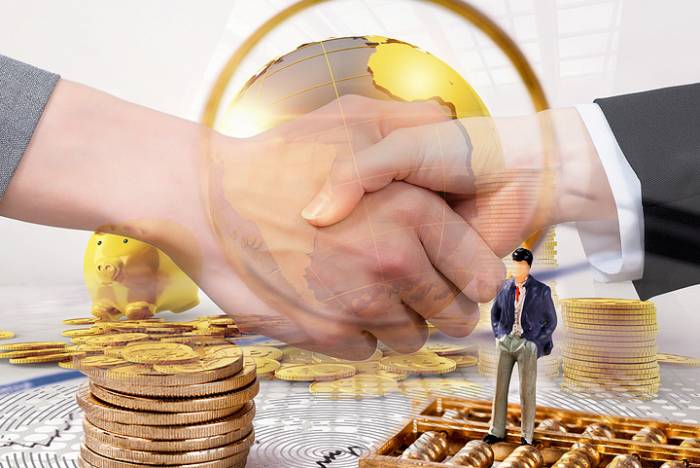
Behind the Surge in Hong Kong IPOs
The acceleration of mainland companies going public in Hong Kong is also significantly propelled by supportive policies. On April 19, the China Securities Regulatory Commission unveiled five measures aimed at improving capital market collaboration with Hong Kong, which included expanding the types of stocks eligible for trading under the Shenzhen-Hong Kong Stock Connect to encompass ETFs and supporting leading domestic firms in pursuing listings in Hong Kong.
Moreover, the regulatory reforms over the years regarding Hong Kong’s capital markets have played a crucial role in attracting large mainland enterprises. For instance, last March, the HKEX amended its Main Board Listing Rules to create dedicated pathways for specialized technology companies, facilitating a smoother entry for these firms.
On September 1, new rules lowering the market capitalization thresholds for companies classified as "specialized technology" went into effect. The minimum thresholds for 'commercialized' and 'non-commercialized' companies were scaled down to HKD 4 billion and HKD 8 billion, respectively. Additionally, in October, HKEX announced optimized timelines for approving initial listing applications, creating fast-track pathways for qualified domestic firms.
According to analysts, the variety of companies from different sectors and at various stages of development choosing Hong Kong for listings indicates a marketplace that is increasingly suited to accommodate various enterprises.
They assert that the ongoing improvements made by Hong Kong's regulatory bodies in the listing mechanism have significantly reduced the costs associated with going public, simplifying the listing process. This enhanced attractiveness for mainland enterprises cannot be overstated.
The influx of influential mainland companies into Hong Kong's market is poised to attract international capital, thereby invigorating and enhancing the appeal of the Hong Kong stock market.
Experts have indicated that as these representative firms from various industries enter the Hong Kong market, local investors will be able to benefit from the dividends associated with China's economic growth. This not only reinforces Hong Kong's stature as an international financial center but also amplifies its global influence.
The Power of Hong Kong's Financial Attraction
The current waves of IPOs have unveiled new prospects in the Hong Kong capital market, significantly aided by the established mutual market connectivity systems.
On November 17, 2024, a milestone was reached with the tenth anniversary of the mutual fund connectivity mechanism between the mainland and Hong Kong capital markets. This system, initiated a decade ago, allows investors to trade shares listed in one market through local brokers in the other, exemplifying a new model of capital market openness.
The Chief Economist of China International Capital Corporation provided insights, stating that from the perspective of the mainland market, this connectivity has facilitated the opening up and internationalization of the capital markets, thus supporting high-level financial interactions. Conversely, in Hong Kong, these mechanisms have broadened the investor base, enhancing market liquidity and depth while improving financial services and overall innovative capabilities.
The HKEX's white paper reflects on the last decade, citing substantial achievements across various dimensions, including product coverage, trading and settlement mechanisms, and investor engagement.
By the end of September 2024, over 3,300 stocks qualified under this connectivity initiative had been added, encompassing over 90% of the total market capitalization and over 80% of transaction volumes across the three markets. The average daily trading volume for Northbound and Southbound trades had skyrocketed compared to the system's initial launch.
Some industry insiders in Hong Kong suggested that it might be worthwhile to consider introducing a similar connectivity model for the primary market, particularly a “New Share Connect” to facilitate IPOs.
"The Hong Kong Exchanges must play a greater role in connecting capital with opportunities, bridging China with the world. Moving forward, our aim is to continually enrich the mutual connectivity products and enhance services to create greater integration within the Hong Kong and mainland financial markets," stated the CEO of HKEX, Nicolas Aguzin.
Comment