Rising Gold Prices
On a bustling Tuesday morning, the financial markets in the United States showcased a vibrant atmosphere, particularly within the precious metals sector. Gold and silver prices surged, capturing the attention of investors, who were closely monitoring the market dynamics influenced by various external factors. A notable decline in the US dollar index lent significant support to the prices of these metals, as they are often inversely correlated with the strength of the dollar. When the dollar dips, gold and silver, priced in the greenback, become comparatively more attractive, showcasing an opportunity for appreciation. In tandem with this, the rise in oil prices echoed the transitions within the global energy market, as the fluctuations reflected an ongoing adjustment in the equilibrium of supply and demand. The relationship between crude oil prices and precious metal sentiments is often intricate, with changes in oil prices capable of impacting investor behavior across the commodity spectrum.
However, amidst the positive trends in gold and silver, a slight uptick in US Treasury yields provided a counterweight to the bullish momentum. As bond yields increase, the appeal of fixed-income securities typically rises, drawing funds away from the more speculative precious metals market. This dynamic contributes to a complex price fluctuation pattern for gold and silver, wherein bullish sentiments and macroeconomic variables converge and collide. For instance, February gold appreciated by $6.60, reaching $2,665.10, while March silver increased by $0.461 to settle at $31.325, depicting a delicate balance yet leaning slightly towards bullishness.
Advertisement
In the broader global arena, Asian and European stock markets experienced a predominantly upward trajectory overnight. When the trading day commenced in New York, the mixed performance of US stock indices suggested a market at a crossroads, coasting near historical highs, buoyed by what is often referred to as the seasonal “Santa Claus Rally.” This phenomenon typically occurs during the last week of December and the first week of January, characterized by an upsurge in stock prices as the year draws to a close, enabling investors to capitalize on positive sentiment and seasonal trends.
As the world of finance continued to evolve, significant political developments in France added an element of uncertainty that investors were keen to navigate. A pivotal no-confidence vote led by Marine Le Pen was slated for Wednesday, stirring concerns over the stability of President Emmanuel Macron's government. Bloomberg reported that French assets had been dragging down European markets since Macron's announcement of snap elections back in June. The distinct divisions seen within the French parliament have hindered decisive action, particularly in addressing the rapidly growing budget deficit. This scenario raised alarms among investors, who found themselves contemplating the implications of a government collapse—a situation that had not been fully priced into financial markets. Michel Barnier, facing the no-confidence vote, highlighted the potential for tumultuous financial repercussions if he were to be dismissed.
As the vote approached, the attention of financial markets and precious metals traders intensified, signifying a moment of high stakes that could fundamentally reshuffle the investment landscape. Coupled with this, the week marked a flurry of economic data anticipated from the United States, culminating in the monthly employment report projected for Friday. Insights from Bloomberg's survey suggested that non-farm payrolls might rise by around 200,000 in November, potentially indicative of a resilient job market.
Federal Reserve Chair Jerome Powell was scheduled to partake in a panel discussion in the midst of a week teeming with economic predictions, including new forecasts from the OECD—a harbinger for shifts in economic policy. Additionally, OPEC's scheduled meeting on Thursday added another layer of intrigue, as speculation swirled around possible adjustments to oil production strategies in response to fluctuating prices.
Within the precious metals sector, external indicators painted a backdrop of a weakening dollar index, further bolstering gold and silver's positions. The price of crude oil futures from the New York Mercantile Exchange hovered around an inviting $69.00 per barrel, while the benchmark 10-year US Treasury yield reached 4.209%, reflecting a competitive landscape where returns on bonds remained attractive alongside the allure of commodities.
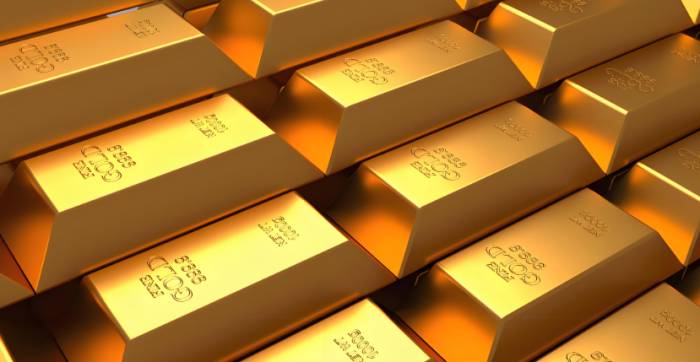
The day’s release of US economic data included multiple reports such as the weekly Johnson Redbook retail sales figures and various indices reflecting consumer sentiment and labor mobility, providing a clearer picture of the economic landscape as participants prepared for the pivotal employment report ahead.
From a technical analysis perspective, it was clear that February bulls in the gold market were maintaining a short-term technical advantage. For bullish traders, the next goal was to establish a solid close above the notable resistance level of $2,748.00, while bears aimed to drive prices down below the solid technical support close to $2,600.00. Resistance levels were anticipated at $2,678.50, followed closely by last Friday’s peak of $2,690.50. On the support side of the equation, $2,650.00 emerged as the first line of defense, with the week’s low of $2,644.50 forming the next layer.
In the silver market, the short-term technical scenario appeared evenly poised between bulls and bears. The bullish faction's next target remains centered on closing prices that exceed the significant resistance marked at $32.50. Conversely, the bearish outlook triggers actions aimed at closing prices below the formidable support level at $30.00. Initial resistance for silver was indicated at the overnight high of $31.49, with subsequent resistance anticipated at $31.75. Support levels were expected to materialize around the overnight low of $30.92, followed by this week’s low at $30.51, as traders vigilantly watched for signs of trend reversals.
Comment